The combined effects of the pandemic on consumer behaviour and economic performance have had profound implications on the acquisition and use of insight.
Organisations are dependent on data and insight to provide the fuel to drive their accelerated digitisation programmes.
Accurate and meaningful predictive insights covering consumer financial and behavioural risk will help organisations navigate the continuing economic uncertainty that is likely to remain for some time.
The predictive insight will help create value by controlling losses and costs. They will also provide the agility to exploit opportunities for increased revenue and growth among those consumers less affected by the pandemic.
Finally, predictive insights will ensure that regulatory and financial reporting and ongoing internal business intelligence are accurate and robust and can be used to shape ongoing organisational strategy.
However, for many organisations, these benefits are yet to be realised. In the age of big data, acquiring insight has become reliant on advanced predictive analytics and machine learning.
While this is widely recognised and understood, only a minority of organisations have successfully leveraged the full value of advanced predictive analytics within their customer, operational and regulatory strategies.
In this series of articles, I’m going to discuss the challenges organisations face in maximising the value of machine learning, and how Experian can help.
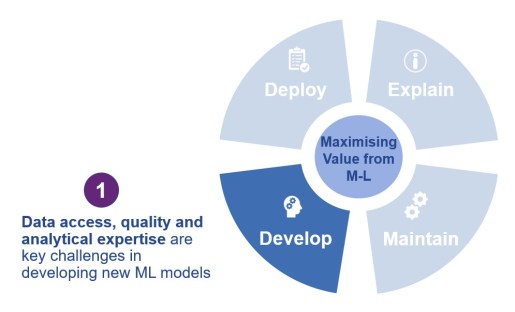
Challenge 1: Data access, data quality and accelerated model development
Research by the World Economic Forum in 2020 found that the top four hurdles in developing machine learning models are quality of data (seen as a hurdle by 91% of respondents), followed by access to talent (84%), access to data (82%), and bias in data (71%).
Ensuring that customer and risk insights are robust, meaningful and differentiated requires access to the widest possible range of traditional and non-traditional data accessed from both internal and external sources.
Data access and data storage can create significant challenges for organisations due to the vast amounts of available data. In addition, internal data may be held across multiple systems and databases and connecting these to a central repository or data lake is a pre-requisite.
Multiple parties will need simultaneous access to this repository.
Data engineers need to build the necessary pipelines to access, cleanse and transform raw data into usable formats that can support the data scientists in developing Machine learning algorithms to drive advanced analytical models.
Often these roles can easily be blurred, and data scientists may be required to support data engineers in preparatory work such as categorising unstructured data into meaningful formats and taxonomies. This means that simultaneous access by multiple users is vital. However, to support compliance and audit requirements for traceability, all data preparation and manipulation activities will need to be tracked and recorded. Designing and building an environment that can simultaneously support and track the requirements of different users can be highly challenging and expensive.
Data scientists are in high demand, and with their skills increasingly being at a premium, they must work efficiently. This will require access to the most up to date modelling tools and programmes to enable them to construct machine learning algorithms. In addition, they need ready access
How Experian can help
Experian’s Ascend Analytics on Demand platform integrates data and analytics into a single scalable, highly secure cloud environment that ensures optimum agility, speed and innovation and which can be accessed simultaneously by multiple users to help accelerate overall model development times.
Ascend’s data staging area creates an intermediate environment for data access, storage and preparation.
It’s designed to help accelerate the ingestion and storage of the widest possible range of traditional and non-traditional data from internal and external sources. This will typically include customer data covering multiple dimensions of identity, credit, transactions, behaviours, preferences and digital devices, plus robust macro-economic forecast data that is sufficiently segmented to reflect sectoral and geographic economic variations.
The integrated analytics environment contains Machine learning-powered categorisation tools to reduce the demands on data scientists.
It frees them to focus on analysing and modelling the data, using different Machine learning algorithms based on the widest possible methodologies, and techniques such as Decision Tree, Random Forest, Gradient Boosting, Neural Networks, Support Vector Machine, and Naïve Bayes.
Ascend supports the rapid, scalable analytical model development, testing, and validation that can facilitate multiple use cases across the organisation.
We can help both new and existing clients to ensure maximum responsiveness to the ongoing post-pandemic economic uncertainty by facilitating increased innovation and faster go-to-market delivery.
Be sure to keep an eye out for my next article in this series, when I will discuss the challenge of deploying machine learning-powered analytical models.