Leandro Guerra
Head of Data Science and Analytics at Experian EMEA and APAC
Advanced analytics can help businesses improve their operations by increasing task automation, reducing human error, and more importantly, enhancing the ability to analyse and interpret vast amounts of data. Research published in Experian’s 2022 Business and Consumer Insight Report found that 62% of companies believe that AI and ML are already radically transforming the way they do business.
Improved business performance translates as the ability to drastically improve the accuracy of models and deliver a more convenient and personalised customer experience, which is of paramount importance in an increasingly digital world. In this article, I will review the main issues involved with the adoption of ML and explore ways that organisations can overcome these challenges.
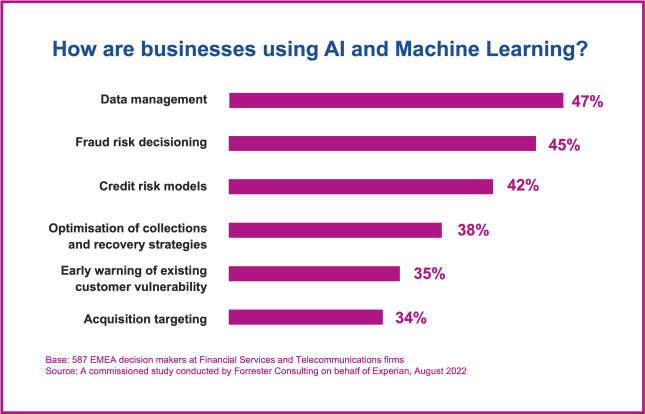
The challenges of advanced analytics
Building the expertise, knowledge and infrastructure necessary to reap the benefits of advanced analytics can pose problems for many businesses. The increased IT complexity required to manage AI and ML was pinpointed as the biggest challenge by 48% of business decision-makers in Experian’s latest research.
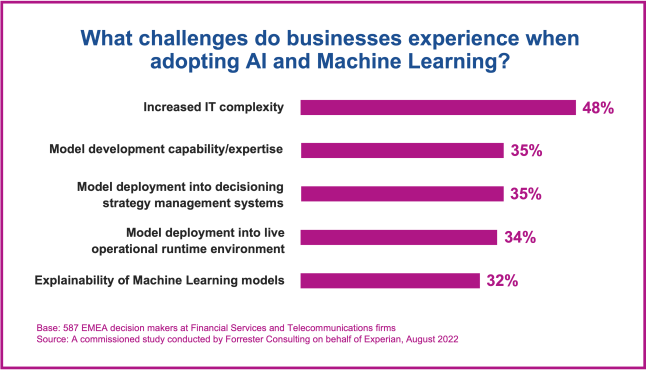
ML Explainability is another key issue that I would like to discuss. As companies accelerate the adoption of advanced analytics, they must ensure the outcomes produced by AI and ML can be understood by those providing regulatory oversight as well as being able to explain to customers why a specific decision has been made.
The proposed European Union AI Act will make explainability mandatory for businesses within the EU and will establish a legal framework for the use of AI in creditworthiness assessments. These regulations are likely to be adopted by other regions in the near future.
In the video below I discuss some of the implications of the AI Act for credit providers.
Our research reveals that almost a third of businesses find the explainability of complex ML models a major challenge. Given the complexity of the processes underlying advanced analytics, ensuring transparency and explainability of ML is not an easy accomplishment – but when done right it can greatly enhance an organisation’s creditworthiness and risk assessment process.
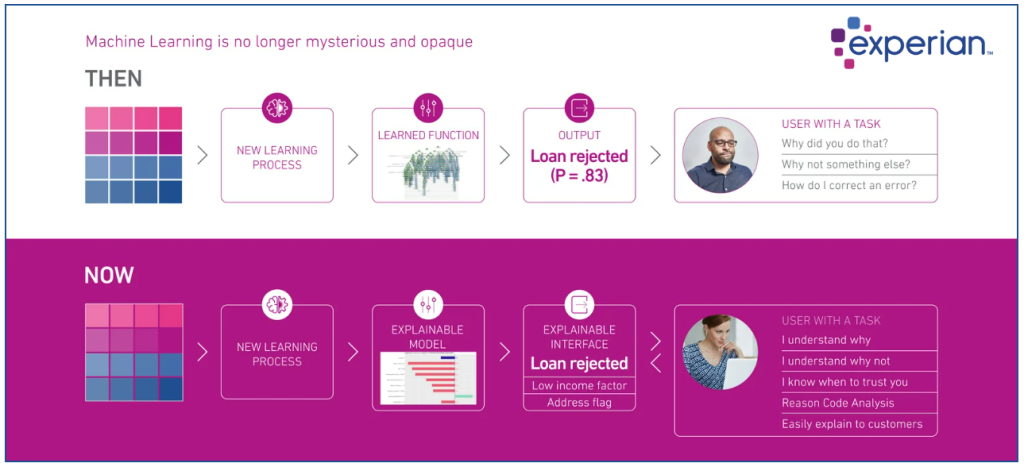
The third key challenge businesses are facing is integration. While AI and ML can support businesses in finding new solutions to problems, integrating them into pre-existing systems is causing headaches for some. Embedding ML requires scalable computing power and sufficient infrastructure to enable it.
Three steps to harness the power of advanced analytics
How can Financial Services and Telecoms providers overcome the challenges of AI and ML to maximise their benefits? Implementing the three tips below is a good place to start.
- Upskilling teams on the multiple facets of advanced analytics is crucial. By upskilling their teams in ML, businesses can ensure they consistently refine the performance of their models and meet regulatory requirements. Currently the gap in IT expertise is one of the main impediments to a wider and more effective adoption of these cutting-edge technologies. Consulting with companies that specialise in AI and ML is often necessary to keep employees up to date, as advanced analytics is constantly evolving. By providing external training organisations can ensure their employees are familiar with the latest advancements in the sector.
- Businesses need to be smart about where to drive investment. Scalable computing power is essential for the effective functioning of ML as it allows businesses to combine huge amounts of data from multiple sources. Cloud-based solutions provide the flexibility to manage and contextualise this data as required, with faster time-to-market for testing and deployment of new credit risk models. Our research reveals that Financial Services and Telecoms providers recognise the importance of this factor. 79% of the companies that have already invested in cloud-based software applications stated that access to more computing power was a major reason for deciding to make such investments.
- The easiest way for companies to take concrete steps towards a better implementation of advanced analytics is by establishing ongoing partnerships with organisations that can provide expertise in AI and ML – both the technology and the regulatory requirements. It can be daunting for any business to master every aspect of advanced analytics. Establishing the right partnership can help them navigate this complex area, complementing and supporting existing analytics teams.
The promise of a new world of opportunities
Overcoming the challenges of advanced analytics will require patience and commitment from Financial Services and Telecoms providers. Yet by leveraging the opportunities that AI and ML provide, they can transform the way they do business and significantly enhance their performance.
Advanced analytics enables businesses to turn large volumes of data into actionable insights. This helps them achieve faster time to market for the testing and deployment of new credit risk and forecasting models. Using these models can significantly improve the accuracy of creditworthiness, affordability, and fraud assessments, which translates into greater revenue.
Businesses that can unleash the full power of advanced analytics will be best placed to reap the rewards. For more insight into the opportunities and challenges involved with AI/ML download Experian’s latest report.